AI in Motor Insurance Inspection: Inspektlabs
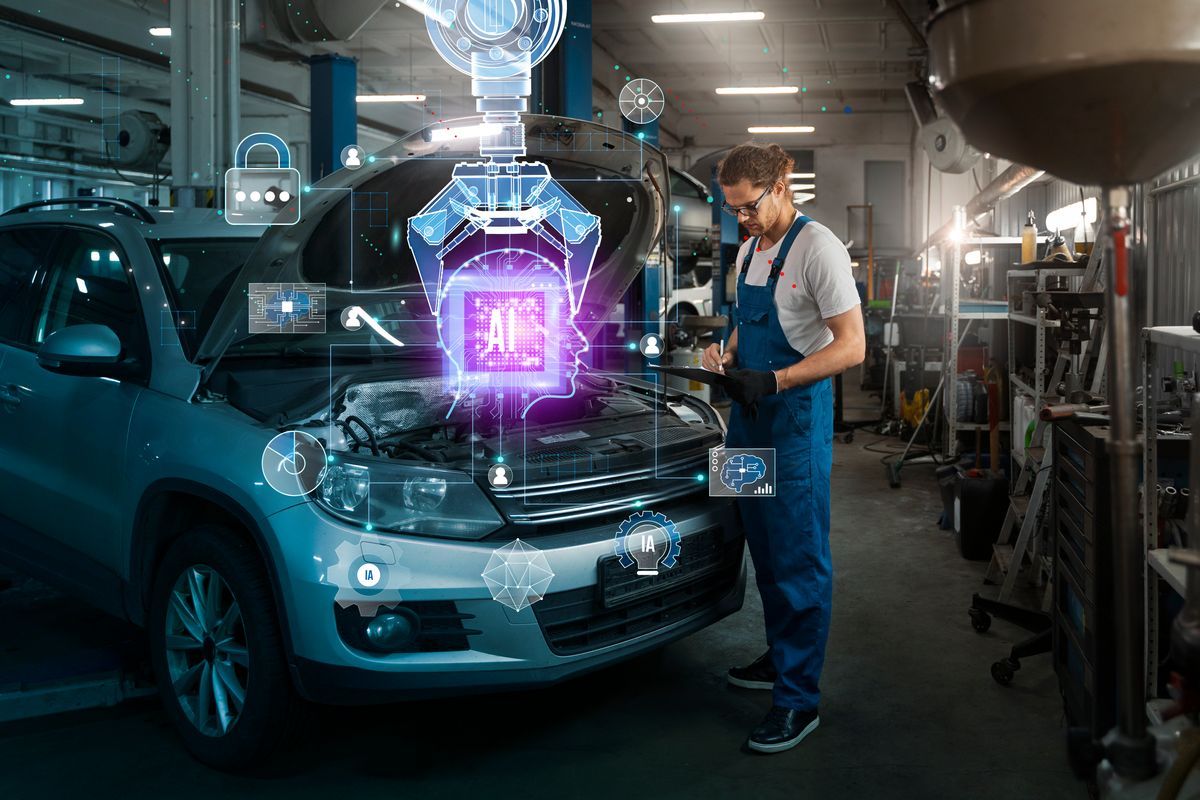
Traditional motor insurance claims are typically hindered by slow and manual inspection processes that rely heavily on human judgment and are susceptible to errors and delays. Insurers often face challenges in accurately assessing vehicle damages and processing claims efficiently, leading to prolonged settlement times and increased operational costs.
AI revolutionizes motor insurance inspections by leveraging advanced algorithms and machine learning capabilities to automate and streamline the inspection process. AI-powered systems can quickly analyze images of vehicle damages, detect patterns, and assess the extent of damage with precision, reducing the dependency on human inspectors and enhancing the accuracy of claim evaluations.
Let's explore how AI transforms the inspection workflow in motor insurance, ensuring faster, more reliable, and cost-effective claim processing.
Inspection Using AI
With AI, the insurance process is divided into four main categories, each optimized to enhance efficiency and accuracy:
- Pre-Inspection
- FNOL (First Notice of Loss)
- Claim Estimation
- Claim Review & subrogration
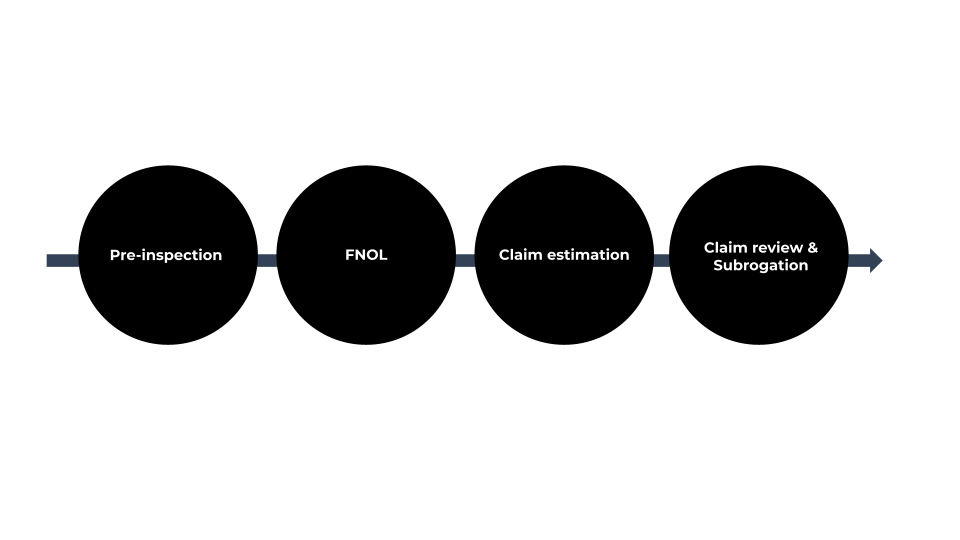
1. Pre-Inspection Using AI
Pre-inspection is a crucial step in the insurance process, conducted to evaluate a vehicle's condition before issuing an insurance policy. The main objectives of pre-inspection are:
- Establishing Baseline Condition: To document the vehicle's current state, capturing any pre-existing damages.
- Preventing Fraud: By recording the vehicle's condition accurately, insurers can prevent fraudulent claims for existing damages being reported as new.
So, how can AI help you in pre-inspection? Let's dive into the transformative power of AI and see how it can streamline the entire process.
How AI Technology Enhances Pre-Inspection Processes
AI-driven pre-inspection solutions are a game-changer. They make the inspection process faster, cheaper, and more accurate. Here's how:
- Efficiency: AI slashes the time required for inspections from days to just minutes.
- Cost-Effectiveness: Automated inspections reduce costs for both insurers and policyholders.
- Accuracy: AI ensures consistent and objective assessments, minimizing human error and the potential for fraud.
There are two main workflows for AI in pre-inspection:
A. Self-Inspection Workflow
- Photo/Video Capture: You, as the vehicle owner, take photos or videos of your car using your smartphone.
- Upload: Simply upload these images or videos to the cloud.
- AI Processing: The Inspektlabs API processes the uploaded media to generate a detailed damage assessment report.
- Policy Decision: Based on the AI-generated report, the insurer makes a policy decision. The possible outcomes are:
- Recommended
- Not Recommended
- Recommended with Declaration
- Refer to Underwriter
The insurer is then notified accordingly.
B. Vendor Inspection Review Workflow
- Inspection: A vendor performs the vehicle inspection and creates a report.
- AI Review: The vendor's report is processed by the Inspektlabs API to flag any high-probability errors.
- Policy Decision: The AI-enhanced report is used to make an informed policy decision, which can be one of the same four outcomes:
- Recommended
- Not Recommended
- Recommended with Declaration
- Refer to Underwriter
Using AI for pre-inspections ensures quicker, more accurate, and reliable vehicle assessments, making the process smooth and hassle-free for both insurers and policyholders.
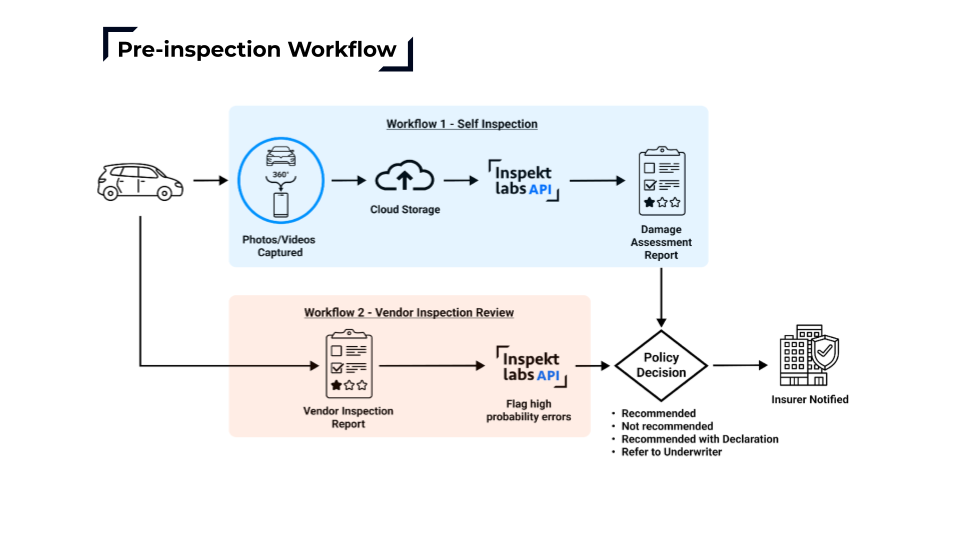
Benefits of Using AI in Pre-Inspection
Utilizing AI in pre-inspection brings numerous benefits, particularly in enhancing fraud detection and ensuring the integrity of insurance claims. Here’s how AI technology improves the process:
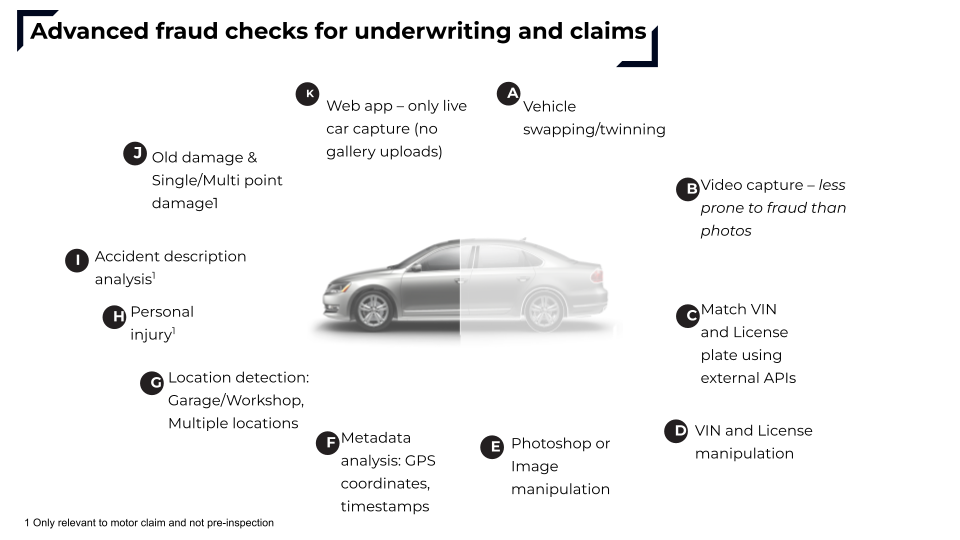
- Automated decision making: AI can not only detect damages, but also take decisions on the policy based on the damages. Insurer provides rules on recommending vs. not recommending a policy based on the damages. Inspektlabs detects damages, and then using Insurer’s rules, takes a decision on the policy
- Vehicle Swapping Detection: AI detects instances where a different vehicle(s) might be used to conceal damages. E.g. a customer captures exterior of one car, and chassis/VIN of a different car
- Location Verification: AI verifies the locations mentioned in claims, such as garages or workshops, to detect any inconsistencies that might suggest fraudulent activities.
- Live Car Capture: By allowing only live captures via a web app, AI ensures that photos of the vehicle are taken in real-time, minimizing the risk of using pre-existing or manipulated images to support false claims.
- Metadata Analysis: AI analyzes metadata such as GPS coordinates and timestamps associated with photos or videos to verify their authenticity, providing insurers with reliable evidence.
- Video Capture Advantage: AI favors video capture over photos, as videos are harder to manipulate, providing insurers with more reliable evidence of the vehicle's condition.
- VIN and License Plate Verification: AI verifies vehicle identification numbers (VIN) and license plates against external databases, ensuring they match and detecting any tampering or manipulation.
- Image Manipulation Detection: AI identifies signs of image manipulation or the use of software like Photoshop to alter evidence, safeguarding insurers against fraudulent claims.
2. First Notice of Loss (FNOL)
Let's delve into the significance of FNOL in the claims process:
What is FNOL?
FNOL, or First Notice of Loss, refers to the initial report made by an insured party to their insurance company immediately after an accident or incident that results in damage to their insured property, such as a vehicle. It is the first step in initiating the claims process.
Importance in the Claims Process
FNOL plays a crucial role in the claims process for several reasons:
- Immediate Action: It prompts insurers to initiate timely responses and assessments, ensuring swift resolution of claims. It allows the insurer to direct the vehicle to the correct location: bodyshop for repairs, and salvage yard for total loss
- Damage Assessment: FNOL enables insurers to promptly assess the extent of damages and determine the appropriate course of action, whether it involves repairs or replacements.
- Customer Service: Providing a seamless FNOL experience enhances customer satisfaction by offering quick support during stressful situations.
- Fraud Prevention: Early reporting allows insurers to investigate claims promptly, mitigating potential fraud and ensuring the integrity of the claims process.
FNOL serves as the foundation for efficient claims handling, facilitating prompt communication between insured parties and insurers to expedite claim settlements and restore normalcy after an unfortunate event.
FNOL should be filed immediately after an accident or incident causing damage to ensure timely assessment and processing of claims.
AI in FNOL:
Using Inspektlabs' API, insurers can efficiently determine whether damaged parts are repairable or need replacement during FNOL:
- Automated Assessment: Inspektlabs' API analyzes images or videos uploaded by the insured to assess the extent of damage.
- Total loss vs Repairable decisions: Based on the damages on the car, Inspektlabs is able to take total loss vs repairable decisions on the vehicle.
- Repair vs. Replace Decision: Based on AI-driven analysis, the API provides recommendations on whether damaged parts can be repaired or require replacement.
- Efficiency: This automated process speeds up claim handling, allowing insurers to make informed decisions swiftly.
- Enhanced Accuracy: AI ensures accurate assessments, minimizing errors in determining the most cost-effective solution for repairs.
Integrating Inspektlabs' AI technology into FNOL enhances efficiency and accuracy, enabling insurers to provide timely and reliable support to their policyholders.
Workflow: Automated Claim Initiation
Customer Interaction: The process begins when a customer reports vehicle damage and receives a notification to capture images.
Image Capture: The customer uses their smartphone to take photos of the damaged vehicle.
Upload Images: The images are uploaded to a cloud storage system.
Cloud Storage and Processing: Stored images are processed to assess the extent of the damage.
Damage Analysis: The system evaluates the damage and determines if further manual review is needed.
Manual Review (if needed): Images requiring additional assessment are sent to an auto manager for evaluation.
3. Claim Estimation Using AI
Let's explore why accurate claim estimation is crucial and the challenges it addresses:
Importance of Accurate Claim Estimation
Accurate claim estimation impacts both financial health and customer service:
- Financial Impact: Ensures insurers allocate appropriate funds, maintaining financial stability.
- Customer Service: Provides fair compensation promptly, enhancing customer satisfaction and loyalty.
Traditional Challenges
Manual estimation poses significant challenges:
- Inaccuracy: Manual processes are prone to errors, leading to inaccurate assessments and potential disputes.
- Time-Consuming: Manual estimations delay claim processing, affecting customer experience and operational efficiency.
Why Accurate Estimation Matters
Accurate estimation is pivotal for:
- Fair Compensation: Ensures policyholders receive appropriate compensation for damages incurred.
- Financial Health: Helps insurers manage claims costs effectively, safeguarding long-term financial sustainability.
Accurate claim estimation, powered by AI, mitigates manual challenges and enhances overall claims management efficiency.
AI Solutions in Claim Estimation
AI claim estimation is a sophisticated application of artificial intelligence aimed at automating and improving the process of assessing vehicle damage and determining repair costs. The primary goal of AI solutions in claim estimation is to streamline the claims process, reduce human error, and enhance overall efficiency for insurance companies and customers alike. AI claim estimation is complex for two reasons:
- Subjectivity: Many decisions in an estimate, such as choosing between repair and replacement, are inherently subjective. The judgment of an adjuster can vary based on experience, interpretation, and even external factors such as the time of day or workload. This subjectivity means that the same adjuster could create two different estimates for the same set of photos taken on different dates. This variability poses a challenge for AI systems, which need to account for and replicate human judgment in a consistent manner.
- Internal Damage: Internal damages, such as those to the radiator or other components not visible from the exterior, are often not captured in customer-submitted photos. These hidden damages can significantly affect the overall repair cost and scope. AI systems primarily relying on visual data from photos might miss these critical aspects, leading to incomplete or inaccurate estimates.
As a result, we automate claim estimation in steps, initially focusing on “single panel minor damage” and gradually expanding to other categories. Below is our approach to AI-based claim estimation:
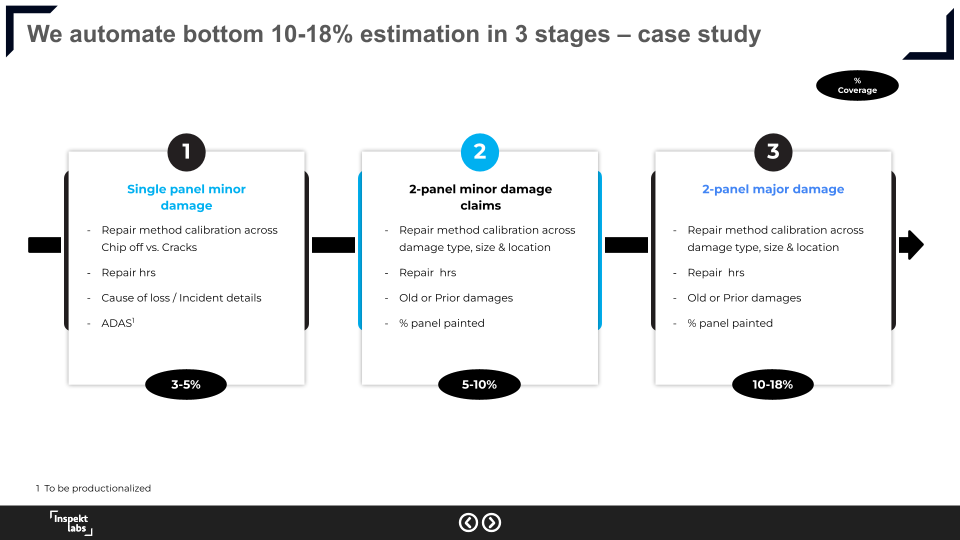
AI revolutionizes claim estimation by enhancing accuracy and efficiency across various damage scenarios:
- Single Panel Minor Damage:
- AI calibrates repair methods for different damage types like chip-offs and cracks.
- Estimates repair hours based on the severity of damage.
- Considers incident details and causes, ensuring precise assessment.
- Factors in ADAS (Advanced Driver Assistance Systems) coverage, typically ranging from 3-5%.
- 2-Panel Minor Damage Claims:
- AI calibrates repair methods based on damage type, size, and location across two panels.
- Accounts for any old or prior damages that may affect repair procedures.
- Calculates the percentage of the panel requiring painting.
- Coverage estimation typically falls between 5-10%.
- 2-Panel Major Damage:
- AI adjusts repair methods for significant damage types, sizes, and locations across two panels.
- Includes considerations for old damages impacting repair complexity.
- Calculates the percentage of panels requiring painting due to damage.
- Coverage estimation varies widely, typically ranging from 10-18%.
AI-driven claim estimation streamlines processes, improves accuracy, and expedites claim settlements, ensuring fair and efficient compensation for policyholders while optimizing insurer operational efficiency.
Claim Review & Subrogation Using AI
Claim review and subrogation is essential to ensure that every claim is treated fairly and consistently. This step guarantees that policyholders receive appropriate compensation based on the actual damage and terms of the policy. It also ensures that insurers apply their policies uniformly, maintaining trust and reliability.
How Inspektlabs Facilitates Claim Subrogation
Accident Occurs: The process starts with a vehicle accident.
Adverse Insurer Notified: The adverse insurer is informed of the accident.
Demand Package Preparation: A demand package is created, including damage photos and a repair estimate.
Insurer Notification and Sharing: The insurer is notified and shares the demand package.
Inspektlabs API: The package is processed through the Inspektlabs API.
AI Subrogation Report: The API generates a report with damage detection, repair value estimation, and high-probability error flagging.
Claim Review and Settlement: The claim is reviewed and settled based on the report. If there are discrepancies, it goes for an exception review before final settlement.
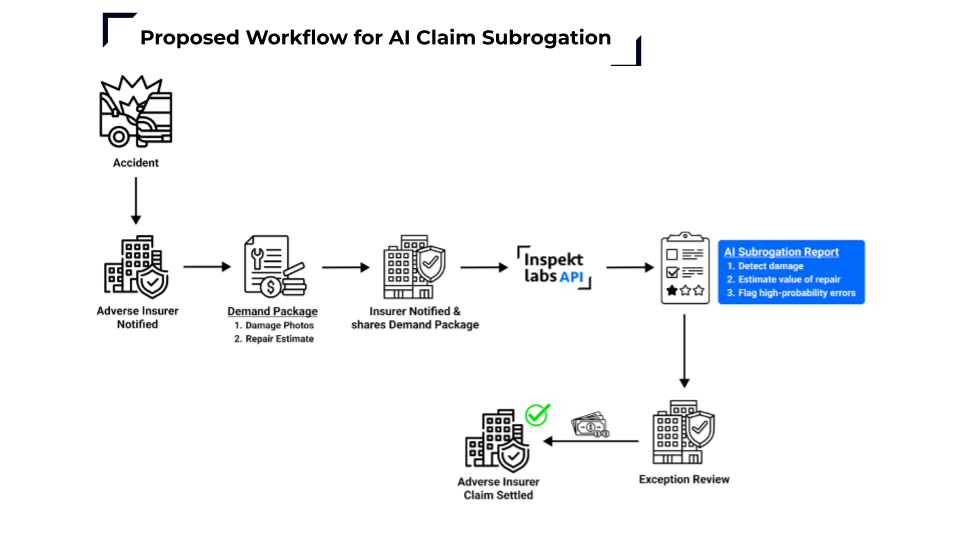
Inspektlabs’ Approach: Claim Review
- Initial Notification - An SMS web link is sent to the customer to start the claim process.
- Video/Photo Capture - The customer captures video or photos of the vehicle damage and uploads them.
- Cloud Storage - The captured media is stored in cloud storage.
- Inspekt Labs API - The stored media is processed through the Inspekt Labs API.
- Claim Assessment - The Inspekt Labs claim assessment includes:
- Repair vs. Replace
- Part price
- Labor cost
- Total loss
- Old damage vs. new repairable
- Delta part mismatch
- Fraud detection
- Review Path - If the claim is straightforward, it goes through the process of account settlement directly. If the claim requires additional review, it is sent to the claims adjuster.
- Claims Adjuster Review - The claims adjuster provides a claim estimate. This estimate may be compared with non-preferred garage estimates.
- Video Rejection or Feedback - If there is an issue with the media captured (e.g., unclear video/photos), the customer receives a notification for video rejection or feedback to provide better media.
- Non-Preferred Garage Review - The comparison of estimates from non-preferred garages and the adjuster’s estimate is made to ensure consistency and accuracy.
- Final Review and Settlement
- Repair vs. replace decision
- Labor cost
- Paint cost
- Potential fraud
- Old damage vs. new damage
After review, if all criteria are met, the claim is settled.
Benefits of AI
Detailed Cases Where AI Improved Claim Review Outcomes AI has significantly improved the claim review process by enhancing accuracy and efficiency. Here are some examples:
- Accident Description Analysis: AI analyzes accident descriptions to ensure they match the visual evidence, identifying discrepancies that may indicate fraud.
- Fraud Detection: AI systems can detect patterns indicative of fraudulent behavior, such as repeated claims for similar damages or inconsistencies in reported incidents.
By leveraging AI, Inspektlabs ensures thorough, accurate, and efficient claim reviews, leading to fair settlements and enhanced customer satisfaction.
Inspektlabs enhances motor insurance inspections by offering faster, more accurate assessments. AI-driven solutions significantly reduce costs and processing times compared to traditional methods. Insurers benefit from improved fraud detection, accurate claim settlements, and increased customer satisfaction. The AI technology ensures consistent and objective evaluations, minimizing human errors and the potential for fraudulent claims.
Future of Motor Insurance Inspection with AI
AI and machine learning technologies are rapidly advancing, promising more sophisticated and accurate vehicle inspections. Innovations such as real-time damage detection, predictive analytics, and enhanced data integration are on the horizon, further improving the efficiency and reliability of insurance processes.
Inspektlabs is continuously evolving, integrating cutting-edge AI technologies to stay ahead in the industry. In the next decade, we can expect further advancements in automated damage assessment, enhanced fraud detection capabilities, and seamless integration with other digital insurance platforms. These developments will ensure more efficient, accurate, and customer-friendly motor insurance inspections.
Conclusion
In summary, we've explored how Inspektlabs revolutionizes motor insurance inspections through AI-driven solutions, enhancing efficiency, accuracy, and fraud detection. From streamlined claim processing to comprehensive pre-inspections and subrogation, Inspektlabs offers significant advantages over traditional methods.
Insurers should consider integrating Inspektlabs' AI technologies to modernize their inspection workflows. By leveraging these innovations, insurers can not only improve operational efficiency but also enhance customer trust and loyalty. Explore how AI can transform your insurance processes and stay ahead in a rapidly evolving industry.
Don't get left behind—embrace the future of auto insurance claims management with Inspektbot. Book a Demo Now !
FAQs
How does AI improve the accuracy of motor insurance inspections? AI improves accuracy by leveraging advanced algorithms to analyze vehicle damage based on objective criteria. This approach reduces reliance on subjective human assessments, ensuring consistent evaluations across inspections. AI also identifies patterns and anomalies in damage reports that may indicate fraud, enhancing overall inspection integrity.
What are the cost benefits of using Inspektlabs' AI solutions? Inspektlabs' AI solutions offer significant cost benefits by reducing the time and resources required for inspections. AI-driven processes streamline the inspection workflow, cutting down on labor costs and inspection times from days to minutes. This efficiency not only lowers operational expenses for insurers but also improves turnaround times for policyholders, enhancing overall customer satisfaction.
How does Inspektlabs ensure the security of uploaded images? Inspektlabs prioritizes data security by employing robust measures throughout the image upload and storage process. Uploaded images are stored in secure cloud environments with encryption protocols that comply with industry standards. This ensures that customer data, including vehicle images and inspection details, remains confidential and protected from unauthorized access or breaches.