Car Inspection Automation: Use-Cases And Complexities | Inspektlabs
As the technology matures further, the feasibility across use-cases is expected to increase significantly and thereby, increasing the adoption of AI based car inspection solutions across use-cases over the next few years.
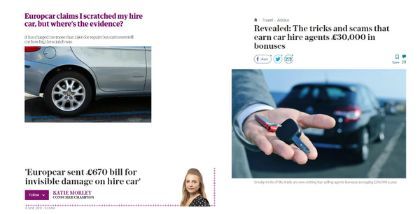
With the rapid expansion of the automobile and insurance industry, it is no wonder that the penetration of AI based solutions for vehicle inspections is growing rapidly. Until recently, traditional human inspection methods has been the only way for car inspections to be carried out. The vehicles must be physically examined by an inspector to determine the extent of the damage and estimate the cost of repairs. Due to human error, there is the possibility that assessments or settlements can be inaccurate in some cases. With the help of machine learning, the car inspection process could be automated, making the process much more convenient for both sides, boosting the productivity of the automotive and insurance players, and increasing customer satisfaction.
Even though this technology has not completely replaced human inspections yet, one can see the clear trajectory of continuous improvement in accuracy of Deep Learning and Computer Vision based systems. Given the limitations of the current technology, we have analyzed several use-cases for car damage assessment using AI to assess feasibility.
What is AI based vehicle inspection?
A Convolution Neural Network model is be the simplest and fastest way to automate such a system, accepting images from a user and determining the damage location and severity. First, the given image must be verified that it is indeed a car image, then it must be confirmed that it is damaged. Those are the entrance checks before we begin the analysis. All gate checks must be validated before a damage check can be conducted. The model will indicate whether the destruction has occurred to the front, side, or rear, as well as its severity whether it was severe, moderate, or minor.
A user input image is processed by the model in five stages:
- Verifies that a given image is of a car.
- Confirms that the car has been damaged.
- Determines whether the damage is on the front, rear, or side
- Determines whether the damage is on certain part e.g. front left door
- Determines whether the damage is minor, moderate, or severe
However, Computer Vision field is still developing and not mature enough to handle all vehicle inspections. Image classification can be easily disrupted by factors like angle, lighting, and resolution. Given this, we have analyzed feasibility of several use-cases for AI based vehicle inspections using photos/videos.
Car Inspection Automation
Increasingly, cars are becoming a shared resource, and are changing ownership more frequently with the advent of micro-insurance and the share economy. Every time a car changes hands, it needs to be inspected. Artificial intelligence can be used to automate 4 primary car inspection processes:
- Motor insurance: Claim assessment and claim review
- Car rental: Damage assessment before & after a journey
- Used car: Damage assessment during the buying/selling process
- Car leasing: Remarketing inspections before the end of the lease
According to the AI-enabled Software-as-a-Service study by IFC and Stellaris Venture Partners, the adoption of an AI solution typically depends on two factors - feasibility and risk.
Compared with other AI use-cases such as radiology and drug recommendation, automobile inspection automation carries relatively lower risks including financial, reputational, and legal risks. On the other hand, the feasibility of car damage assessment generally depends on the use case. In the following section, we have explored several use-cases and have allocated feasibility scores to each use-case based on the current state of AI technology. However, as the technology matures, the feasibility for each use-case will only increase over time.
Motor Insurance
Use-cases
Automated claim assessment or repair value estimation of a damaged car is a strong use case for insurers. This would not only help in reducing the assessment costs but also improve the overall customer experience with a quicker settlement of claims.
Feasibility challenges
- Internal damages: Medium and High severity accidents cause significant internal damage which is not visible in external photos/videos. Even experienced adjustors cannot assess internal damage using external photos/videos.
- Repair estimation complexities: Repair estimation not only requires damage assessment but a deep understanding of claim estimation and access to OEM data e.g., part costs, labor costs, etc. Inspektlabs has partnered with several providers of OEM data across the world to generate repair estimates customized for each market
- Subjectivity: In addition to this, repair estimates are highly subjective. A single assessor can create 2 different repair estimates for the same car at different times.
Feasibility score
7/10
Claim estimation for small claims: These will be typically fender benders having external damage only, or external damage with minor internal damage.
9/10
Claim review for all claims covering subjective decisions on external panels e.g. a part that could have been repaired was replaced, 5 hrs of labor was charged when only 1 hr of labor was required.
Car Rental
Use case
- Damage assessment before & after the journey
- Customers often feel cheated when they are charged for damages by rental companies
- Car inspection automation can provide a trustworthy and transparent platform for vehicle inspections
Feasibility challenges
- Customer incentive to hide damages: The customer has an incentive to hide damages during drop-off. As a result, the customer may capture photos/videos to hide smaller damages to the car. Inspektlabs has built technology to provide real-time feedback to customers during the photo/video capture. (see link: https://inspektlabs.com/blog/360-video-solution-for-car-damage-assessment-with-ai-2/). This makes it very difficult for the customer to hide damages during the drop-off
Feasibility score
8/10
Used car
Use-case
Damage assessment during the buying/selling process using photos/videos
Feasibility challenges
- Micro-damages: For used-car buying and selling, micro-damages are quite important. Micro-damages are damages that are not visible from a 2-3 ft distance. Given even humans cannot identify such damages from a 2-3 ft distance, we need highly zoom-in views for such damages (see images below)
- Engine and repainting evaluation: In addition to this, while buying or selling a car, one needs to evaluate the engine and repainting as well. These 2 factors have a significant impact on the car valuation. However, these 2 factors cannot be resolved with computer vision-based solutions.
Feasibility score
7/10
- A human inspector would be required for assessing engines, repainting, and micro-damages. However, AI-based solutions can be used to improve the accuracy of the assessment and make the process faster.
- Inspeklabs has specialized web apps and APIs that focus on augmenting the human inspector (instead of replacing the human inspector).
Car leasing
Use-case
End of lease inspections. At the end of the lease, the car needs to be inspected for renewal /termination of the lease.
Feasibility challenges
In case the customer wants to terminate the lease, a very thorough car inspection may be required wherein micro-damages, engine, and repainting are evaluated (similar to used car use-case)
Feasibility score
9/10
Focus on inspections conducted by customers for renewal of lease only (and not termination). As a result, micro-damages are not required to be covered.
The Bigger Picture
Adoption of an AI solution typically depends on two factors - feasibility and risk. For car inspection automation using AI, risks are much lower than other AI applications such as radiology. The risks associated with AI based car inspections are primarily financial risks.
The feasibility of AI based car inspection solutions can be evaluated across use-cases, ranging from motor insurance claims to used car inspection automation. Certain use-cases such as automation of large claims has technological feasibility challenges, and therefore, to increase the adoption of AI solutions, Inspektlabs focuses on high feasibility use-cases e.g., small motor claim automation, augmenting human inspector for used car inspection etc.
As the technology matures further, the feasibility across use-cases is expected to increase significantly and thereby, increasing the adoption of AI based car inspection solutions across use-cases over the next few years.