Leveraging AI to Detect Internal Damages for Vehicle Inspection | Inspektlabs
Detecting internal damages accurately is extremely important for reliable damage inspection reports. Inaccurate reports will lead to incorrect insurance premiums and, more importantly, they will present a considerable safety risk.
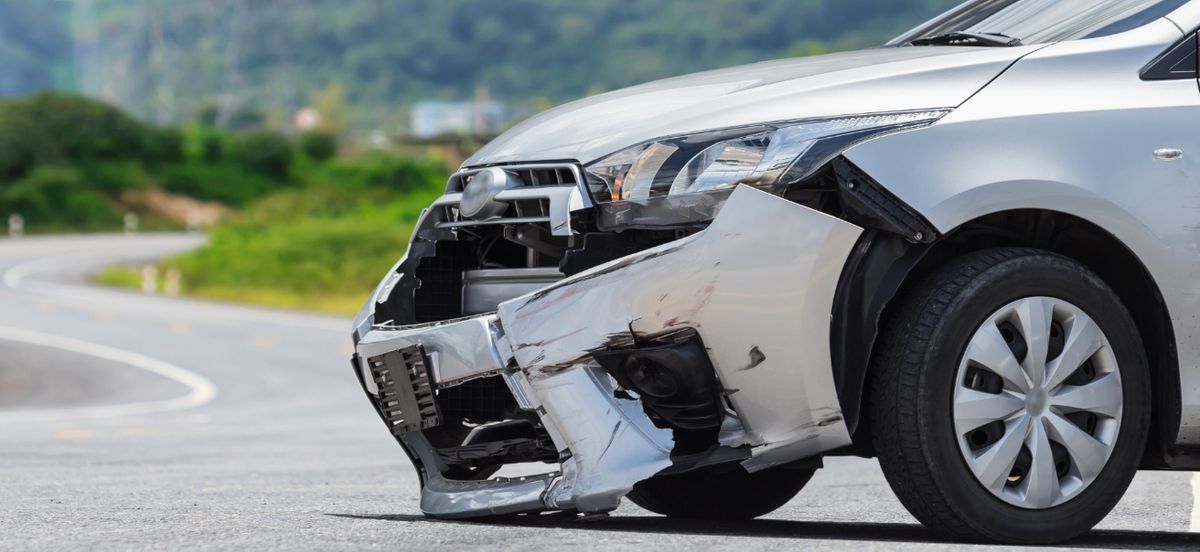
Introduction
Detecting car damages through AI is more effective than manual inspections. The damage reports generated by AI are highly reliable and take just a few seconds. Detecting damage to a car has two primary facets, i.e., internal damages and external damages. So far, vehicle damage detection using AI has been limited to external damages that are clearly visible in a photo or a video. While detecting external damages is comparatively straightforward, internal damages present an entirely unique challenge. Even experienced adjustors cannot gauge internal damages through this approach. The complication arises because internal damages are not visible in photos and videos. Due to this, many internal damages can go undetected, resulting in inaccurate inspection reports.
For example, the image below clearly shows that the front bumper has sustained damage. However, it is still challenging to ascertain whether the corresponding internal parts such as the beam, condenser, radiator, cooling fan, and engine have also sustained damage or not.
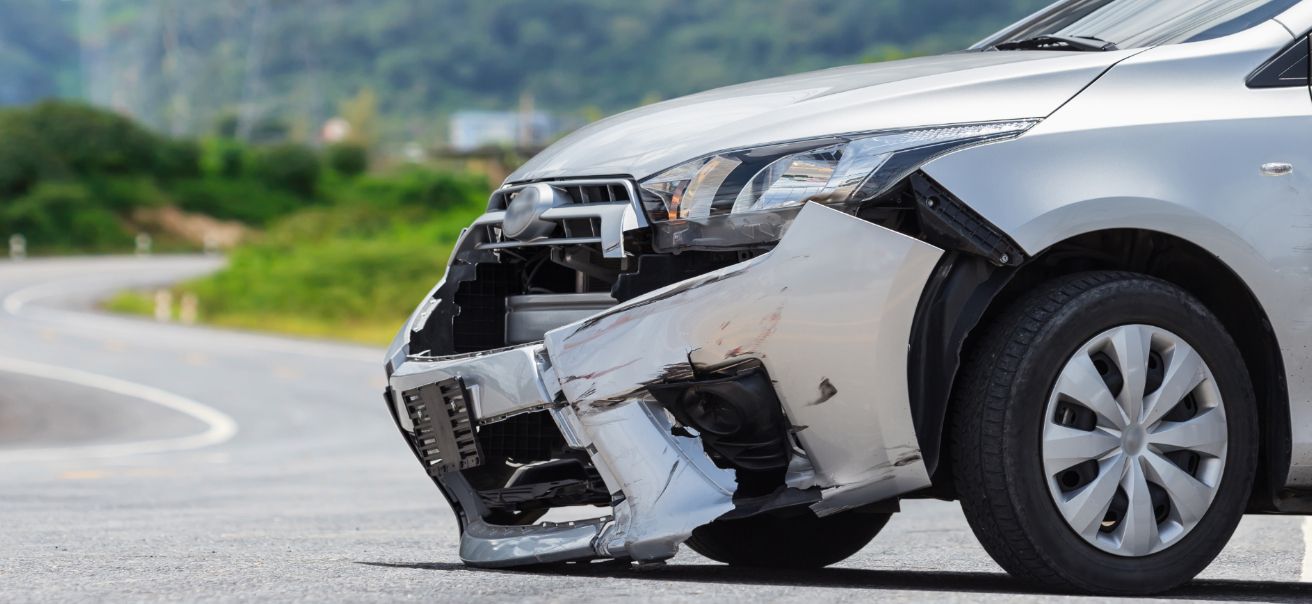
Inspektlabs has solved this problem using a two-pronged approach. The following two methods allow us to analyze the internal damages sustained by a car.
1. Dismantled Parts
The first method is by inspecting the photos or videos of dismantled parts. In some scenarios, like when it comes to brake discs and brake pads, AI damage detection does prove to be helpful through the dismantled parts approach. For example, the brake disc becomes visible after removing the wheel and can therefore be assessed for damages using photos and videos. Naturally, capturing photos/videos of dismantled parts is not the most feasible approach in most instances. The customer cannot be expected to dismantle the car to capture the photos and videos of the damaged parts. If the car does need to be dismantled, it requires significant time and effort to undertake the process. At that point, conducting a manual inspection will be more feasible because the dismantled parts can be assessed quickly.
Nonetheless, this approach has utility in specific cases. Inspektlabs' AI can inspect such parts within seconds and generate a comprehensive report. Let us discuss how this damage inspection with AI works.
By analyzing photos/videos of the dismantled parts, the algorithm can detect what part it is, the type of damage, and the severity of the damage (% value). Based on these factors, it also suggests a solution such as a repair or replacement. It further predicts the number of hours it would take to follow through with the proposed solution. For example, the AI has detected damages in the car part shown below.
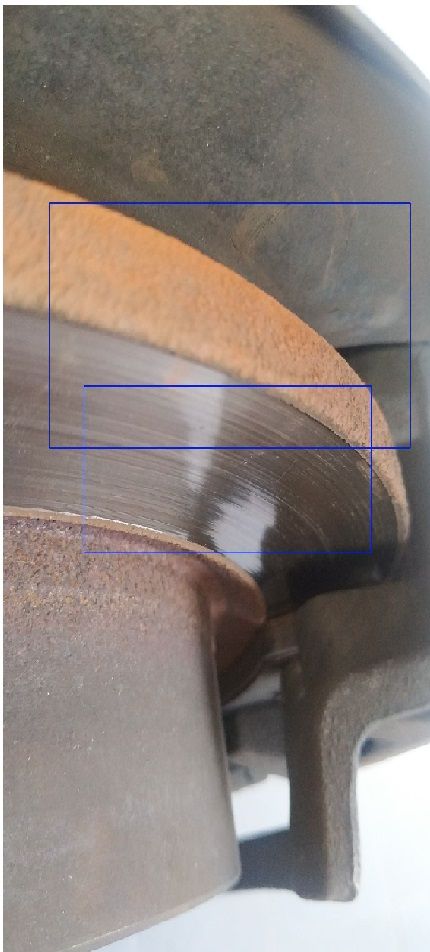
After analyzing the image, the AI generated the following summarized damage report.

The AI successfully identified the part as a brake disc. It further classified the damage as 'scratch or spot' and identified the presence of rust. The AI has judged the severity of the damage as 79% and therefore recommended that the part be replaced. According to its estimate, the total replacement time for this part will take 0.5 hours. Let us consider another example. The AI has marked up the damages on the part depicted in the image below.
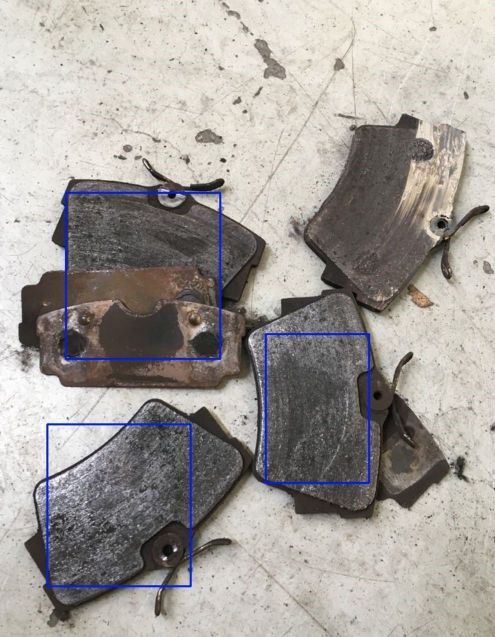
After analyzing the image, the AI generated the following summarized damage report.

The AI successfully identified the part as a brake pad. It further classified the damage as 'worn out.' The AI has judged the severity of the damage as 100% and therefore recommended that the part be replaced. According to the estimate, the total labor required for replacing this part is 0.5 hours.
2. Predicting Internal Damages based on the Scope and Extent of External Damages
In cases where the first approach does not work, we use the alternative approach, which involves predicting internal damages by assessing the external damages. Generally, the extent of the internal damages depends on the depth of the external damages. If the external damage is deep, there is a higher probability that there will be internal damage. For example, if there's a dent at the bottom center of the tailgate, then the tailgate lock presents a high probability of damage. Similarly, if there is significant damage to the front bumper, then there is an extremely high likelihood that the radiator is damaged.
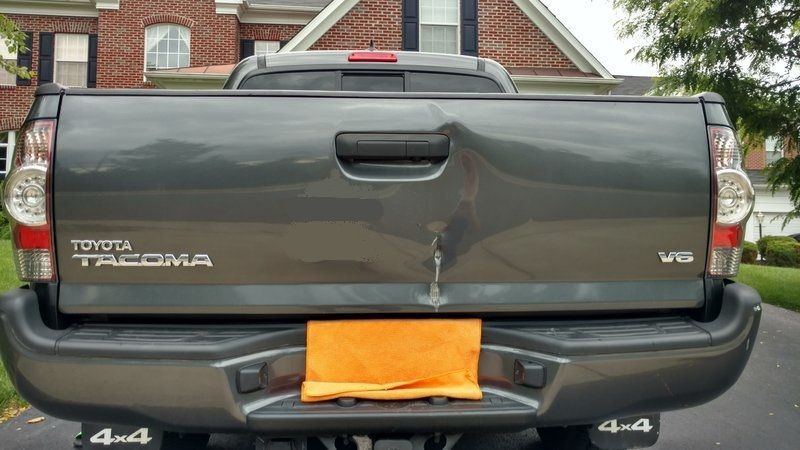
The AI functions by first identifying the parts that are detectable. Then, it lists the damages that each part has sustained and assigns a damage severity score accordingly. It suggests the recommended solution, such as replacement or repair, and also identifies whether the part had sustained prior damage. The inspection report also estimates the amount of time required to carry out the suggested solution. This analysis is done for every detectable part.
Based on the external damages that the AI detects, it determines and lists which internal parts have a high probability of damage. The AI also assigns a confidence score, which serves as a reliable indicator of the likelihood of damage. Again, it suggests a potential solution, such as repair or replacement, based on its assessment. There are two levels to this approach.
Level 1: The Generic Car Model Approach
The first approach, which we have discussed so far, relies on the generic structure of the car. This approach works effectively for the majority of cars. Let us consider a few examples to understand how it works. In the image below, the car's hood has clearly sustained damage. If we follow the generic structure of a car, the following internal parts correspond to the car's hood: bonnet lock, hood insulator, hood hinges, and headlight bracket. Therefore, if there is damage to the hood, these internal parts have a higher likelihood of sustaining damage.
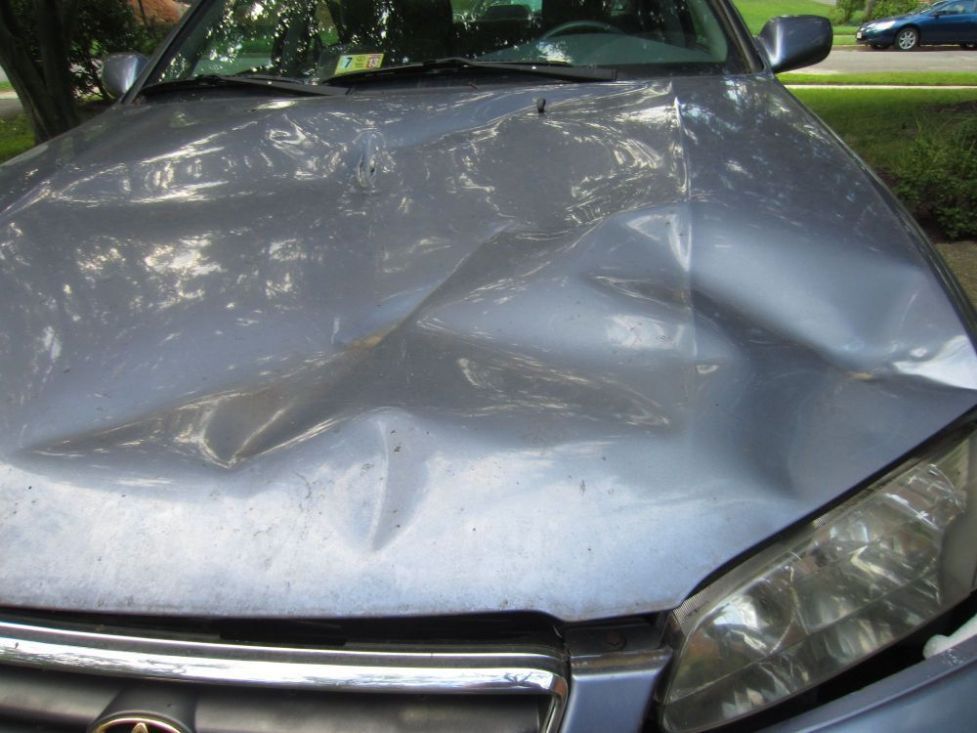
Similarly, consider the image below. The back bumper has sustained significant damage. Therefore, there is a probability that the corresponding interior parts like the rear beam, sensor, back panel, qtr lining, and rear PDC sensor may also be damaged.
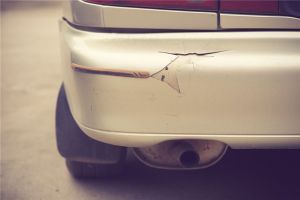
In the image below, the left rear door has clearly sustained damage. Therefore, the following corresponding internal parts present a likelihood of sustaining damage: the left rear door inner handle, left rear door latch, left rear door lock, left rear door pad, and the speakers.
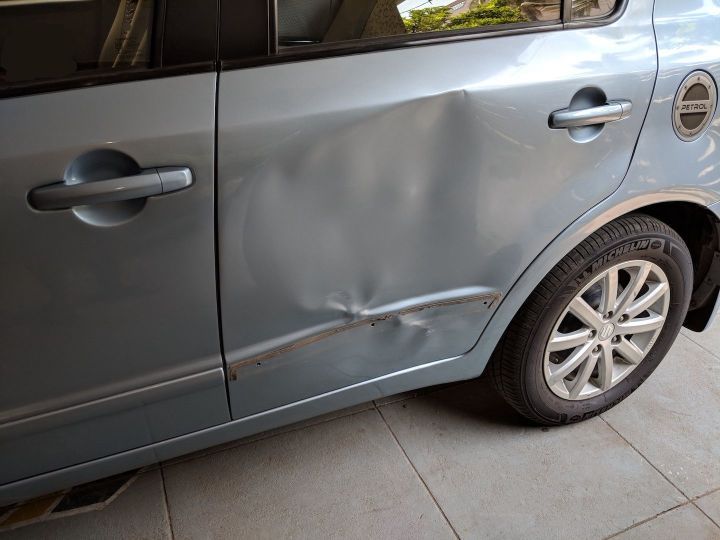
In all the examples presented above, the AI can successfully detect and identify the scope and extent of the external damages. Then, based on its analysis, the algorithm will generate a report which predicts the probability of damages to the corresponding internal parts. Such AI damage inspections standardize and streamline vehicle damage detection. However, this is not a one-size-fits-all solution for every car since there can be many variations in car structures. For example, electric cars do not follow the generic structure and necessitate a different approach.
Level 2: The 3D Car Model Approach
The generic structure approach is inadequate when dealing with electric cars or other cars with an atypical structure. This is because the external parts will likely not have the same corresponding internal parts in such cars. In such cases, a 3D model of the car's interior can help assess the extent and scope of internal damages. Assessing internal damages through 3D models is a new and innovative technology that is still being developed.
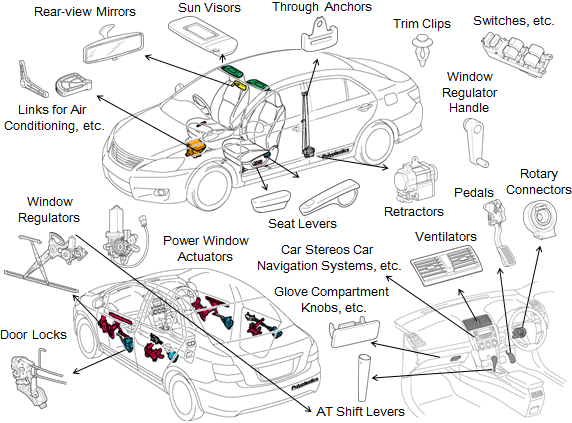
There are numerous advantages to this approach. First, it helps inspect all those cars that follow an atypical structure. Second, if we have access to the 3D model of a car, the AI will generate a highly accurate and reliable inspection report. Third, this approach affords great flexibility because the AI can process the unique 3D models for every vehicle. Although this approach is under development, it offers excellent potential that will further enhance the capabilities of damage inspection with AI.
Conclusion
Detecting internal damages accurately is extremely important for reliable damage inspection reports. Inaccurate reports will lead to incorrect insurance premiums and, more importantly, they will present a considerable safety risk. If internal damages are not assessed accurately, they may cause accidents or cause further damage to other parts of the car. Internal damage detection through AI is highly reliable and devoid of human error. The AI can successfully predict internal damages through dismantled parts and also through external damage estimations.